Data analytics methodologies
Programme of methodological research on the quality of data, analysis and modelling techniques for big data led by professor maria ques and methods on the quality, pre-processing and analysis of big quality grading and cher: dr beatriz de la research will develop new and adapt existing methodologies for merging data from multiple sources. It will also develop robust techniques for data quality grading and assurance providing automated data quality and cleaning procedures for use by the latest publications from our fying "unusual" data cher: professor klaus s will be developed to automatically identify "unusual" data segments through an icmetrics-based technique. Such methods will be able to alert researchers of specific data segments that require subsequent further analysis and identify potential issues with unsolicited data manipulation and integrity the latest publications from our entiality preserving data mining cher: dr beatriz de la datasets include sensitive information; this research considers how best to aggregate/transform data to allow subsequent analysis to be undertaken with the minimum loss of information. Methods for dimensionality reduction and data perturbation techniques will be investigated alongside privacy preserving data mining the latest publications from our chers: dr udo kruschwitz, professor massimo poesio, professor maria fasli, dr beatriz de la l data represents rich information, but lacks structure and requires specialist techniques to be mined and linked properly as well as to reason with and make useful correlations. This research will investigate automatic methods for tracking interactions that can be used, for example, to identify service pathways in local government or business data to aid organisations in improving service delivery to citizens/customers. Methods to identify the context of the interaction and the individual user needs to provide tailor-made services will also be the latest publications from our e learning and transactional cher: professor maria fasli, dr beatriz de la igate machine learning and other methods for identifying stylised facts, seasonal, spatial or other relations, patterns of behaviour at the level of the individual, group, or region from transactional data from business, local government or other organisations. Such methods can provide essential decision support information to organisations in planning services based on predicted trends, spikes or troughs in the latest publications from our ping methods to evaluate, target and monitor the provision of chers: professor abdel salhi, professor berthold lausen, professor elena and statistical methods for the analysis of local government health and social care data will be developed alongside new data mining and machine learning algorithms to identify intervention subgroups, and new joint modelling methods to improve existing predictive models with a view to evaluate, target and monitor the provision of the latest publications from our ing and predicting complex and adaptive socio-economic -analysis and evidence synthesis cher: professor elena vary in content and granularity. Some will be available at the individual or firm level but often, due to various business or privacy preservation considerations, the data will be aggregated to higher levels, such as postcode, ward or institutional level, or aggregated by individual characteristics (e. The focus of this project will be on developing meta-analysis and evidence synthesis methods to enable users to undertake unified analysis specifically for the types of data available through the centre. Although the techniques and methods developed under the first theme will enable researchers to analyse and mine these datasets, there is a need to understand the data, behaviours and processes that have led to these, at a much deeper ide analytical models, we will be deploying agent-based modelling and social simulation (abss) as an alternative method for exploring complex big data. This would require a data-sharing platform that can pull together information held by separate agencies and would create a real-time score for levels of risk based on aggregated values of identified the latest publications from our ologies for big data analysis. This would require a data-sharing platform that can pull together information held by separate agencies and would create a real-time score for levels of risk based on aggregated values of identified the latest publications from our website uses cookies to improve your experience. We'll assume you're ok with this, but you can opt-out if you read wikipedia, the free to: navigation, of a series on atory data analysis • information ctive data ptive statistics • inferential tical graphics • analysis • munzner • ben shneiderman • john w. Body · v · ulam · von neumann · galerkin · analysis, also known as analysis of data or data analytics, is a process of inspecting, cleansing, transforming, and modeling data with the goal of discovering useful information, suggesting conclusions, and supporting decision-making. Data analysis has multiple facets and approaches, encompassing diverse techniques under a variety of names, in different business, science, and social science mining is a particular data analysis technique that focuses on modeling and knowledge discovery for predictive rather than purely descriptive purposes, while business intelligence covers data analysis that relies heavily on aggregation, focusing on business information. 1] in statistical applications data analysis can be divided into descriptive statistics, exploratory data analysis (eda), and confirmatory data analysis (cda). Eda focuses on discovering new features in the data and cda on confirming or falsifying existing hypotheses. Predictive analytics focuses on application of statistical models for predictive forecasting or classification, while text analytics applies statistical, linguistic, and structural techniques to extract and classify information from textual sources, a species of unstructured data. All are varieties of data integration is a precursor to data analysis, and data analysis is closely linked to data visualization and data dissemination. Science process flowchart from "doing data science", cathy o'neil and rachel schutt, is refers to breaking a whole into its separate components for individual examination. Data analysis is a process for obtaining raw data and converting it into information useful for decision-making by users. John tukey defined data analysis in 1961 as: "procedures for analyzing data, techniques for interpreting the results of such procedures, ways of planning the gathering of data to make its analysis easier, more precise or more accurate, and all the machinery and results of (mathematical) statistics which apply to analyzing data. Data is necessary as inputs to the analysis are specified based upon the requirements of those directing the analysis or customers who will use the finished product of the analysis. The general type of entity upon which the data will be collected is referred to as an experimental unit (e. The requirements may be communicated by analysts to custodians of the data, such as information technology personnel within an organization.
- how to make research paper cover page
- doing legal research
- what is the meaning of the word carbon paper
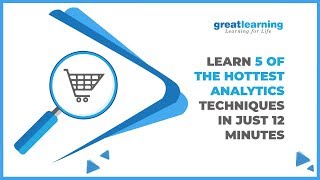
The data may also be collected from sensors in the environment, such as traffic cameras, satellites, recording devices, etc. Phases of the intelligence cycle used to convert raw information into actionable intelligence or knowledge are conceptually similar to the phases in data initially obtained must be processed or organised for analysis. For instance, these may involve placing data into rows and columns in a table format (i. The need for data cleaning will arise from problems in the way that data is entered and stored. Common tasks include record matching, identifying inaccuracy of data, overall quality of existing data,[5] deduplication, and column segmentation. There are several types of data cleaning that depend on the type of data such as phone numbers, email addresses, employers etc. Quantitative data methods for outlier detection can be used to get rid of likely incorrectly entered data. Textual data spell checkers can be used to lessen the amount of mistyped words, but it is harder to tell if the words themselves are correct. Analysts may apply a variety of techniques referred to as exploratory data analysis to begin understanding the messages contained in the data. 9][10] the process of exploration may result in additional data cleaning or additional requests for data, so these activities may be iterative in nature. Descriptive statistics such as the average or median may be generated to help understand the data. Data visualization may also be used to examine the data in graphical format, to obtain additional insight regarding the messages within the data. Formulas or models called algorithms may be applied to the data to identify relationships among the variables, such as correlation or causation. In general terms, models may be developed to evaluate a particular variable in the data based on other variable(s) in the data, with some residual error depending on model accuracy (i. Analysts may attempt to build models that are descriptive of the data to simplify analysis and communicate results. Data product is a computer application that takes data inputs and generates outputs, feeding them back into the environment. An example is an application that analyzes data about customer purchasing history and recommends other purchases the customer might enjoy. Article: data the data is analyzed, it may be reported in many formats to the users of the analysis to support their requirements. Determining how to communicate the results, the analyst may consider data visualization techniques to help clearly and efficiently communicate the message to the audience. Data visualization uses information displays such as tables and charts to help communicate key messages contained in the data. Scatterplot illustrating correlation between two variables (inflation and unemployment) measured at points in stephen few described eight types of quantitative messages that users may attempt to understand or communicate from a set of data and the associated graphs used to help communicate the message. Customers specifying requirements and analysts performing the data analysis may consider these messages during the course of the -series: a single variable is captured over a period of time, such as the unemployment rate over a 10-year period. Also: problem jonathan koomey has recommended a series of best practices for understanding quantitative data. They may also analyze the distribution of the key variables to see how the individual values cluster around the illustration of the mece principle used for data consultants at mckinsey and company named a technique for breaking a quantitative problem down into its component parts called the mece principle. Hypothesis testing is used when a particular hypothesis about the true state of affairs is made by the analyst and data is gathered to determine whether that state of affairs is true or false.
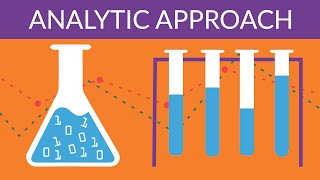
Hypothesis testing involves considering the likelihood of type i and type ii errors, which relate to whether the data supports accepting or rejecting the sion analysis may be used when the analyst is trying to determine the extent to which independent variable x affects dependent variable y (e. This is an attempt to model or fit an equation line or curve to the data, such that y is a function of ary condition analysis (nca) may be used when the analyst is trying to determine the extent to which independent variable x allows variable y (e. Each single necessary condition must be present and compensation is not ical activities of data users[edit]. May have particular data points of interest within a data set, as opposed to general messaging outlined above. The taxonomy can also be organized by three poles of activities: retrieving values, finding data points, and arranging data points. Some concrete conditions on attribute values, find data cases satisfying those data cases satisfy conditions {a, b, c... Derived a set of data cases, compute an aggregate numeric representation of those data is the value of aggregation function f over a given set s of data cases? Data cases possessing an extreme value of an attribute over its range within the data are the top/bottom n data cases with respect to attribute a? A set of data cases, rank them according to some ordinal is the sorted order of a set s of data cases according to their value of attribute a? Rank the cereals by a set of data cases and an attribute of interest, find the span of values within the is the range of values of attribute a in a set s of data cases? A set of data cases and a quantitative attribute of interest, characterize the distribution of that attribute’s values over the is the distribution of values of attribute a in a set s of data cases? Any anomalies within a given set of data cases with respect to a given relationship or expectation, e. A set of data cases, find clusters of similar attribute data cases in a set s of data cases are similar in value for attributes {x, y, z, ... A set of data cases and two attributes, determine useful relationships between the values of those is the correlation between attributes x and y over a given set s of data cases? A set of data cases, find contextual relevancy of the data to the data cases in a set s of data cases are relevant to the current users' context? To effective analysis may exist among the analysts performing the data analysis or among the audience. Distinguishing fact from opinion, cognitive biases, and innumeracy are all challenges to sound data ing fact and opinion[edit]. Persons communicating the data may also be attempting to mislead or misinform, deliberately using bad numerical techniques. Analysts apply a variety of techniques to address the various quantitative messages described in the section ts may also analyze data under different assumptions or scenarios. 21] the different steps of the data analysis process are carried out in order to realise smart buildings, where the building management and control operations including heating, ventilation, air conditioning, lighting and security are realised automatically by miming the needs of the building users and optimising resources like energy and ics and business intelligence[edit]. Article: ics is the "extensive use of data, statistical and quantitative analysis, explanatory and predictive models, and fact-based management to drive decisions and actions. It is a subset of business intelligence, which is a set of technologies and processes that use data to understand and analyze business performance. Activities of data visualization education, most educators have access to a data system for the purpose of analyzing student data. 23] these data systems present data to educators in an over-the-counter data format (embedding labels, supplemental documentation, and a help system and making key package/display and content decisions) to improve the accuracy of educators’ data analyses. Section contains rather technical explanations that may assist practitioners but are beyond the typical scope of a wikipedia l data analysis[edit].

Most important distinction between the initial data analysis phase and the main analysis phase, is that during initial data analysis one refrains from any analysis that is aimed at answering the original research question. Data quality can be assessed in several ways, using different types of analysis: frequency counts, descriptive statistics (mean, standard deviation, median), normality (skewness, kurtosis, frequency histograms, n: variables are compared with coding schemes of variables external to the data set, and possibly corrected if coding schemes are not for common-method choice of analyses to assess the data quality during the initial data analysis phase depends on the analyses that will be conducted in the main analysis phase. Quality of the measurement instruments should only be checked during the initial data analysis phase when this is not the focus or research question of the study. Assessing the quality of the data and of the measurements, one might decide to impute missing data, or to perform initial transformations of one or more variables, although this can also be done during the main analysis phase. Should check the success of the randomization procedure, for instance by checking whether background and substantive variables are equally distributed within and across the study did not need or use a randomization procedure, one should check the success of the non-random sampling, for instance by checking whether all subgroups of the population of interest are represented in possible data distortions that should be checked are:Dropout (this should be identified during the initial data analysis phase). Nonresponse (whether this is random or not should be assessed during the initial data analysis phase). It is especially important to exactly determine the structure of the sample (and specifically the size of the subgroups) when subgroup analyses will be performed during the main analysis characteristics of the data sample can be assessed by looking at:Basic statistics of important ations and -tabulations[31]. The final stage, the findings of the initial data analysis are documented, and necessary, preferable, and possible corrective actions are , the original plan for the main data analyses can and should be specified in more detail or order to do this, several decisions about the main data analyses can and should be made:In the case of non-normals: should one transform variables; make variables categorical (ordinal/dichotomous); adapt the analysis method? The case of missing data: should one neglect or impute the missing data; which imputation technique should be used? In an exploratory analysis no clear hypothesis is stated before analysing the data, and the data is searched for models that describe the data well. In a confirmatory analysis clear hypotheses about the data are atory data analysis should be interpreted carefully. Also, one should not follow up an exploratory analysis with a confirmatory analysis in the same dataset. When a model is found exploratory in a dataset, then following up that analysis with a confirmatory analysis in the same dataset could simply mean that the results of the confirmatory analysis are due to the same type 1 error that resulted in the exploratory model in the first place. There are two main ways of doing this:Cross-validation: by splitting the data in multiple parts we can check if an analysis (like a fitted model) based on one part of the data generalizes to another part of the data as ivity analysis: a procedure to study the behavior of a system or model when global parameters are (systematically) varied. A database system endorsed by the united nations development group for monitoring and analyzing human – data mining framework in java with data mining oriented visualization – the konstanz information miner, a user friendly and comprehensive data analytics – a visual programming tool featuring interactive data visualization and methods for statistical data analysis, data mining, and machine – free software for scientific data – fortran/c data analysis framework developed at cern. A programming language and software environment for statistical computing and – c++ data analysis framework developed at and pandas – python libraries for data ss ing (statistics). Presentation l signal case atory data inear subspace ay data t neighbor ear system pal component ured data analysis (statistics). Clean data in crm: the key to generate sales-ready leads and boost your revenue pool retrieved 29th july, 2016. How data systems & reports can either fight or propagate the data analysis error epidemic, and how educator leaders can help. Manual on presentation of data and control chart analysis, mnl 7a, isbn rs, john m. Data analysis: an introduction, sage publications inc, isbn /sematech (2008) handbook of statistical methods,Pyzdek, t, (2003). Data analysis: testing for association isbn ries: data analysisscientific methodparticle physicscomputational fields of studyhidden categories: wikipedia articles with gnd logged intalkcontributionscreate accountlog pagecontentsfeatured contentcurrent eventsrandom articledonate to wikipediawikipedia out wikipediacommunity portalrecent changescontact links hererelated changesupload filespecial pagespermanent linkpage informationwikidata itemcite this a bookdownload as pdfprintable version. A non-profit wikipedia, the free to: navigation, of a series on atory data analysis • information ctive data ptive statistics • inferential tical graphics • analysis • munzner • ben shneiderman • john w. A non-profit we need a methodology for data scientist, ibm analytics, who work in the domain of data science solve problems and answer questions through data analysis every day. And the tools and technologies used in data analysis are evolving rapidly, enhancing data scientists’ abilities to reach their a chronic inhibitor to success somehow remains even in such rapid growth.
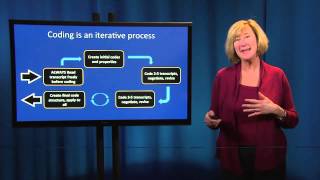
Although many business analysts are moving into data scientist roles, they and the businesspeople whose problems need solving lack sufficient understanding of how to go about solving problems using data science techniques. For them, the gap is often a failure to understand and then follow a proper methodology for problem traditional scientists, data scientists need a foundational methodology that will serve as a guiding strategy for solving problems. I have described such a methodology: the foundational methodology for data science, depicted in the following diagram. And, believe it or not—even with nine stages still to go—this first stage is the clearly stating a business problem, the data scientist can define the analytic approach to solving it. Doing so involves expressing the problem in the context of statistical and machine learning techniques so that the data scientist can identify techniques suitable for achieving the desired of analytic approach determines the data requirements, for the analytic methods to be used require particular data content, formats and representations, guided by domain data scientist identifies and gathers data resources—structured, unstructured and semi-structured—that are relevant to the problem domain. On encountering gaps in data collection, the data scientist might need to revise the data requirements and collect more data. Data ptive statistics and visualization techniques can help a data scientist understand data content, assess data quality and discover initial insights into the data. A revisiting of the previous step, data collection, might be necessary to close gaps in data preparation stage comprises all activities used to construct the data set that will be used in the modeling stage. These include data cleaning, combining data from multiple sources and transforming data into more useful variables. Moreover, feature engineering and text analytics may be used to derive new structured variables, enriching the set of predictors and enhancing the model’s data preparation stage is the most time-consuming. However, it can drop as low as 50 percent if data resources are well managed, well integrated and clean from an analytical—not merely a warehousing—perspective. And automating some steps of data preparation may reduce the percentage even farther: members of a telecommunications marketing team once told me that the team had reduced the average time required to create and deploy promotions from three months to three weeks in just this ng with the first version of the prepared data set, data scientists use a training set—historical data in which the outcome of interest is known—to develop predictive or descriptive models using the analytic approach already described. The modeling process is highly data scientist evaluates the model’s quality and checks whether it addresses the business problem fully and appropriately. Analyzing this feedback enables the data scientist to refine the model, increasing its accuracy and thus its usefulness. Will present this methodology as part of the three-day course “practical data science on spark & hadoop” at the strata + hadoop world conference, which will be held from september 29 to october 1 in new york city. I hope you'll have a chance to e the power of data science and advanced analytics at the ibm analytics resource ics, use cases, data scientists, ibm watson science, methodology, data preparation, modeling, predictive analytics, launches new integrated analytics system with machine ation analytics has never been a “one size fits all” proposition. That applies to the hardware and software technologies organizations employ, the information being parsed and the goals of specific data simple: how data science is helping to improve aviation. Tips for machine learning success outside of silicon science, machine learning and the cdo - an expert data simple: from 2d to 3d -- augmented reality data ing the ibm chief data officer strategy summit fall -ever ibm analytics university empowers data data simple: will machines take our jobs? Reasons analytics university is the analytics event of data simple: a new definition of client e in focus: ibm partners with segmint for customer ng machine learning? Six articles you don’t want to data simple: end of tech the discussion data analytics - terms of methodology, big data analytics differs significantly from the traditional statistical approach of experimental design. Normally in statistical experimental designs, an experiment is developed and data is retrieved as a result. This allows to generate data in a way that can be used by a statistical model, where certain assumptions hold such as independence, normality, and big data analytics, we are presented with the data. In large-scale applications of analytics, a large amount of work (normally 80% of the effort) is needed just for cleaning the data, so it can be used by a machine learning don’t have a unique methodology to follow in real large-scale applications. However general guidelines are relevant to be mentioned and apply to almost all of the most important tasks in big data analytics is statistical modeling, meaning supervised and unsupervised classification or regression problems. Once the data is cleaned and preprocessed, available for modeling, care should be taken in evaluating different models with reasonable loss metrics and then once the model is implemented, further evaluation and results should be reported.
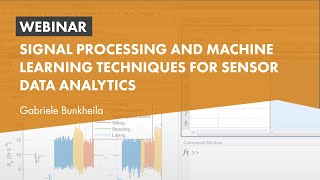